In physics, a Langevin equation (named after Paul Langevin) is a stochastic differential equation describing how a system evolves when subjected to a combination of deterministic and fluctuating ("random") forces. The dependent variables in a Langevin equation typically are collective (macroscopic) variables changing only slowly in comparison to the other (microscopic) variables of the system. The fast (microscopic) variables are responsible for the stochastic nature of the Langevin equation. One application is to Brownian motion, which models the fluctuating motion of a small particle in a fluid.
Brownian motion as a prototype
The original Langevin equation[1][2] describes Brownian motion, the apparently random movement of a particle in a fluid due to collisions with the molecules of the fluid,
Here, is the velocity of the particle, is its damping coefficient, and is its mass. The force acting on the particle is written as a sum of a viscous force proportional to the particle's velocity (Stokes' law), and a noise term representing the effect of the collisions with the molecules of the fluid. The force has a Gaussian probability distribution with correlation function
where is Boltzmann's constant, is the temperature and is the i-th component of the vector . The -function form of the time correlation means that the force at a time is uncorrelated with the force at any other time. This is an approximation: the actual random force has a nonzero correlation time corresponding to the collision time of the molecules. However, the Langevin equation is used to describe the motion of a "macroscopic" particle at a much longer time scale, and in this limit the -correlation and the Langevin equation becomes virtually exact.
Another common feature of the Langevin equation is the occurrence of the damping coefficient in the correlation function of the random force, which in an equilibrium system is an expression of the Einstein relation.
Mathematical aspects
A strictly -correlated fluctuating force is not a function in the usual mathematical sense and even the derivative is not defined in this limit. This problem disappears when the Langevin equation is written in integral form
Therefore, the differential form is only an abbreviation for its time integral. The general mathematical term for equations of this type is "stochastic differential equation".
Another mathematical ambiguity occurs for Langevin equations with multiplicative noise, which refers to noise terms that are multiplied by a non-constant function of the dependent variables, e.g., . If a multiplicative noise is intrinsic to the system, its definition is ambiguous, as it is equally valid to interpret it according to Stratonovich- or Ito- scheme (see Itō calculus). Nevertheless, physical observables are independent of the interpretation, provided the latter is applied consistently when manipulating the equation. This is necessary because the symbolic rules of calculus differ depending on the interpretation scheme. If the noise is external to the system, the appropriate interpretation is the Stratonovich one.[3][4]
Generic Langevin equation
There is a formal derivation of a generic Langevin equation from classical mechanics.[5][6] This generic equation plays a central role in the theory of critical dynamics,[7] and other areas of nonequilibrium statistical mechanics. The equation for Brownian motion above is a special case.
An essential step in the derivation is the division of the degrees of freedom into the categories slow and fast. For example, local thermodynamic equilibrium in a liquid is reached within a few collision times, but it takes much longer for densities of conserved quantities like mass and energy to relax to equilibrium. Thus, densities of conserved quantities, and in particular their long wavelength components, are slow variable candidates. This division can be expressed formally with the Zwanzig projection operator.[8] Nevertheless, the derivation is not completely rigorous from a mathematical physics perspective because it relies on assumptions that lack rigorous proof, and instead are justified only as plausible approximations of physical systems.
Let denote the slow variables. The generic Langevin equation then reads
The fluctuating force obeys a Gaussian probability distribution with correlation function
This implies the Onsager reciprocity relation for the damping coefficients . The dependence of on is negligible in most cases. The symbol denotes the Hamiltonian of the system, where is the equilibrium probability distribution of the variables . Finally, is the projection of the Poisson bracket of the slow variables and onto the space of slow variables.
In the Brownian motion case one would have , or and . The equation of motion for is exact: there is no fluctuating force and no damping coefficient .
Examples
Thermal noise in an electrical resistor
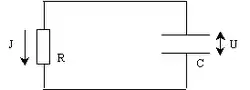
There is a close analogy between the paradigmatic Brownian particle discussed above and Johnson noise, the electric voltage generated by thermal fluctuations in a resistor.[9] The diagram at the right shows an electric circuit consisting of a resistance R and a capacitance C. The slow variable is the voltage U between the ends of the resistor. The Hamiltonian reads , and the Langevin equation becomes
This equation may be used to determine the correlation function
which becomes white noise (Johnson noise) when the capacitance C becomes negligibly small.
Critical dynamics
The dynamics of the order parameter of a second order phase transition slows down near the critical point and can be described with a Langevin equation.[7] The simplest case is the universality class "model A" with a non-conserved scalar order parameter, realized for instance in axial ferromagnets,
Other universality classes (the nomenclature is "model A",..., "model J") contain a diffusing order parameter, order parameters with several components, other critical variables and/or contributions from Poisson brackets.[7]
Harmonic oscillator in a fluid
A particle in a fluid is described by a Langevin equation with a potential energy function, a damping force, and thermal fluctuations given by the fluctuation dissipation theorem. If the potential is quadratic then the constant energy curves are ellipses, as shown in the figure. If there is dissipation but no thermal noise, a particle continually loses energy to the environment, and its time-dependent phase portrait (velocity vs position) corresponds to an inward spiral toward 0 velocity. By contrast, thermal fluctuations continually add energy to the particle and prevent it from reaching exactly 0 velocity. Rather, the initial ensemble of stochastic oscillators approaches a steady state in which the velocity and position are distributed according to the Maxwell–Boltzmann distribution. In the plot below (figure 2), the long time velocity distribution (orange) and position distributions (blue) in a harmonic potential () is plotted with the Boltzmann probabilities for velocity (red) and position (green). In particular, the late time behavior depicts thermal equilibrium.
Trajectories of free Brownian particles
Consider a free particle of mass with equation of motion described by
where is the particle velocity, is the particle mobility, and is a rapidly fluctuating force whose time-average vanishes over a characteristic timescale of particle collisions, i.e. . The general solution to the equation of motion is
where is the correlation time of the noise term. It can also be shown that the autocorrelation function of the particle velocity is given by[10]
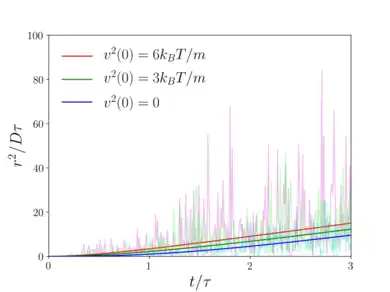
where we have used the property that the variables and become uncorrelated for time separations . Besides, the value of is set to be equal to such that it obeys the equipartition theorem. If the system is initially at thermal equilibrium already with , then for all , meaning that the system remains at equilibrium at all times.
The velocity of the Brownian particle can be integrated to yield its trajectory . If it is initially located at the origin with probability 1, then the result is
Hence, the average displacement asymptotes to as the system relaxes. The mean squared displacement can be determined similarly:
This expression implies that , indicating that the motion of Brownian particles at timescales much shorter than the relaxation time of the system is (approximately) time-reversal invariant. On the other hand, , which indicates an irreversible, dissipative process.
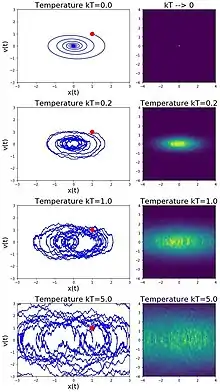
Recovering Boltzmann statistics
If the external potential is conservative and the noise term derives from a reservoir in thermal equilibrium, then the long-time solution to the Langevin equation must reduce to the Boltzmann distribution, which is the probability distribution function for particles in thermal equilibrium. In the special case of overdamped dynamics, the inertia of the particle is negligible in comparison to the damping force, and the trajectory is described by the overdamped Langevin equation
where is the damping constant. The term is white noise, characterized by (formally, the Wiener process). One way to solve this equation is to introduce a test function and calculate its average. The average of should be time-independent for finite , leading to
Itô's lemma for the Itô drift-diffusion process says that the differential of a twice-differentiable function f(t, x) is given by
Applying this to the calculation of gives
This average can be written using the probability density function ;
where the second term was integrated by parts (hence the negative sign). Since this is true for arbitrary functions , it follows that
thus recovering the Boltzmann distribution
Turbulent Kinetic Energy in the Atmospheric Surface Layer
The probability density function p(k) of the turbulent kinetic energy k is investigated for diabatic atmospheric surface layer (ASL) flows.[11] In the latter reference, authors show when the velocity components are near-Gaussian and their squared amplitudes are nearly independent, the resulting p(k) is shown to be γ-distributed. therefore, a nonlinear Langevin equation that preserves a γ-distributed p(k), but allows linear relaxation of k to its mean state, is proposed and tested using multiple ASL data sets.
Equivalent techniques
In some situations, one is primarily interested in the noise-averaged behavior of the Langevin equation, as opposed to the solution for particular realizations of the noise. This section describes techniques for obtaining this averaged behavior that are distinct from—but also equivalent to—the stochastic calculus inherent in the Langevin equation.
Fokker–Planck equation
A Fokker–Planck equation is a deterministic equation for the time dependent probability density of stochastic variables . The Fokker–Planck equation corresponding to the generic Langevin equation described in this article is the following:[12]
The equilibrium distribution is a stationary solution.
Klein–Kramers equation
The Fokker–Planck equation for an underdamped Brownian particle is called the Klein–Kramers equation.[13][14] If the Langevin equations are written as
where is the momentum, then the corresponding Fokker–Planck equation is
Here and are the gradient operator with respect to r and p, and is the Laplacian with respect to p.
In -dimensional free space, corresponding to on , this equation can be solved using Fourier transforms. If the particle is initialized at with position and momentum , corresponding to initial condition , then the solution is[14][15]
where
In three spatial dimensions, the mean squared displacement is
Path integral
A path integral equivalent to a Langevin equation can be obtained from the corresponding Fokker–Planck equation or by transforming the Gaussian probability distribution of the fluctuating force to a probability distribution of the slow variables, schematically . The functional determinant and associated mathematical subtleties drop out if the Langevin equation is discretized in the natural (causal) way, where depends on but not on . It turns out to be convenient to introduce auxiliary response variables . The path integral equivalent to the generic Langevin equation then reads[16]
where is a normalization factor and
The path integral formulation allows for the use of tools from quantum field theory, such as perturbation and renormalization group methods.
See also
References
- ↑ Langevin, P. (1908). "Sur la théorie du mouvement brownien [On the Theory of Brownian Motion]". C. R. Acad. Sci. Paris. 146: 530–533.
- ↑ Lemons, Don S.; Gythiel, Anthony (1997). "Paul Langevin's 1908 paper "On the Theory of Brownian Motion" ["Sur la théorie du mouvement brownien," C. R. Acad. Sci. (Paris) 146, 530–533 (1908)]". American Journal of Physics. American Association of Physics Teachers (AAPT). 65 (11): 1079–1081. Bibcode:1997AmJPh..65.1079L. doi:10.1119/1.18725. ISSN 0002-9505.
- ↑ van Kampen, N. G. (1981). "Itô versus Stratonovich". Journal of Statistical Physics. Springer Science and Business Media LLC. 24 (1): 175–187. Bibcode:1981JSP....24..175V. doi:10.1007/bf01007642. ISSN 0022-4715. S2CID 122277474.
- ↑ Stochastic Processes in Physics and Chemistry. Elsevier. 2007. doi:10.1016/b978-0-444-52965-7.x5000-4. ISBN 978-0-444-52965-7.
- ↑ Kawasaki, K. (1973). "Simple derivations of generalized linear and nonlinear Langevin equations". J. Phys. A: Math. Nucl. Gen. 6 (9): 1289–1295. Bibcode:1973JPhA....6.1289K. doi:10.1088/0305-4470/6/9/004.
- ↑ Dengler, R. (2015). "Another derivation of generalized Langevin equations". arXiv:1506.02650v2 [physics.class-ph].
- 1 2 3 Hohenberg, P. C.; Halperin, B. I. (1977). "Theory of dynamic critical phenomena". Reviews of Modern Physics. 49 (3): 435–479. Bibcode:1977RvMP...49..435H. doi:10.1103/RevModPhys.49.435. S2CID 122636335.
- ↑ Zwanzig, R. (1961). "Memory effects in irreversible thermodynamics". Phys. Rev. 124 (4): 983–992. Bibcode:1961PhRv..124..983Z. doi:10.1103/PhysRev.124.983.
- ↑ Johnson, J. (1928). "Thermal Agitation of Electricity in Conductors". Phys. Rev. 32 (1): 97. Bibcode:1928PhRv...32...97J. doi:10.1103/PhysRev.32.97.
- ↑ Pathria RK (1972). Statistical Mechanics. Oxford: Pergamon Press. pp. 443, 474–477. ISBN 0-08-018994-6.
- ↑ Allouche, Mohammad; Katul, Gabriel G.; Fuentes, Jose D.; Bou-Zeid, Elie (1 July 2021). "Probability law of turbulent kinetic energy in the atmospheric surface layer". Physical Review Fluids. 6 (7): 074601. doi:10.1103/PhysRevFluids.6.074601. S2CID 237743132.
- ↑ Ichimaru, S. (1973), Basic Principles of Plasma Physics (1st. ed.), USA: Benjamin, p. 231, ISBN 0805387536
- ↑ Kramers, H.A. (1940). "Brownian motion in a field of force and the diffusion model of chemical reactions". Physica. Elsevier BV. 7 (4): 284–304. Bibcode:1940Phy.....7..284K. doi:10.1016/s0031-8914(40)90098-2. ISSN 0031-8914. S2CID 33337019.
- 1 2 Risken, H. (1989). The Fokker–Planck Equation: Method of Solution and Applications. New York: Springer-Verlag. ISBN 978-0387504988.
- ↑ Chandrasekhar, S. (1943). "Stochastic Problems in Physics and Astronomy". Reviews of Modern Physics. 15 (1): 1–89. Bibcode:1943RvMP...15....1C. doi:10.1103/RevModPhys.15.1. ISSN 0034-6861.
- ↑ Janssen, H. K. (1976). "Lagrangean for Classical Field Dynamics and Renormalization Group Calculations of Dynamical Critical Properties". Z. Phys. B. 23 (4): 377–380. Bibcode:1976ZPhyB..23..377J. doi:10.1007/BF01316547. S2CID 121216943.
Further reading
- W. T. Coffey (Trinity College, Dublin, Ireland) and Yu P. Kalmykov (Université de Perpignan, France, The Langevin Equation: With Applications to Stochastic Problems in Physics, Chemistry and Electrical Engineering (Third edition), World Scientific Series in Contemporary Chemical Physics - Vol 27.
- Reif, F. Fundamentals of Statistical and Thermal Physics, McGraw Hill New York, 1965. See section 15.5 Langevin Equation
- R. Friedrich, J. Peinke and Ch. Renner. How to Quantify Deterministic and Random Influences on the Statistics of the Foreign Exchange Market, Phys. Rev. Lett. 84, 5224 - 5227 (2000)
- L.C.G. Rogers and D. Williams. Diffusions, Markov Processes, and Martingales, Cambridge Mathematical Library, Cambridge University Press, Cambridge, reprint of 2nd (1994) edition, 2000.