拟蒙特卡罗方法
数值分析中,拟蒙特卡罗方法(Quasi-Monte Carlo method)是使用低差异列(一种确定生成的超均匀分布列,也称为拟随机列、次随机列)来进行数值积分和研究其它一些数值问题的方法。而普通的蒙特卡罗方法或蒙地卡罗积分方法使用的是伪随机数。MATLAB中提供了生成如哈尔顿列、索博尔列等超均匀分布列的函数[1]。
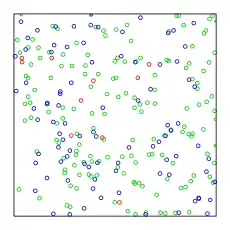
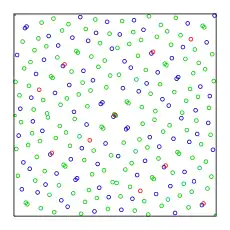
拟蒙特卡罗方法和蒙特卡罗方法的具体内容相似,要解决的问题都是通过测量某个可测函数 f 在某些点上的取值,而在数值上求它的积分的近似值。例如要求在单位体积上的积分近似,可以设取的点为x1, ..., xN,那么:
其中的xi都是s维向量。拟蒙特卡罗方法和普通蒙特卡罗方法的区别在于xi的具体选取方式。蒙特卡罗方法用的是伪随机列,而拟蒙特卡罗方法用到的是哈尔顿列、索博尔列等低差异列。使用低差异列的优点是收敛速率较快。拟蒙特卡罗方法可以达到O(1/N)的收敛速率,而普通蒙特卡罗方法的收敛速率则是 O(N-0.5)[2]。
近年来,拟蒙特卡罗方法在金融数学和计算机数学领域里得到了越来越多的应用[2],因为其中常常会需要计算高维积分的数值近似。蒙特卡罗方法和拟蒙特卡罗方法可以快捷简单地得到较好的结果。
误差估计
拟蒙特卡罗方法的近似误差可以用取点x1, ..., xN的差异度作为上限。具体来说,Koksma-Hlawka不等式表明,误差项
被
限制,其中V(f)为函数f的Hardy-Krause变差[3],DN是(x1,...,xN)的差异度,定义为
- ,
其中Q是任何[0,1]s中边界与坐标轴平行的方形“块”[3]。表明拟蒙特卡罗方法的近似误差大约是的量级,于此相对的是普通蒙特卡罗方法的近似误差为量级。注意这里的不等式给出的是误差上限,事实上拟蒙特卡罗方法的收敛速率要比其上限所示的速率快得多[2]。因此,一般来说拟蒙特卡罗方法比起普通的蒙特卡罗方法来说大大加快了收敛的速率。
参考来源
- Generating Quasi-Random Numbers
- Søren Asmussen and Peter W. Glynn, Stochastic Simulation: Algorithms and Analysis, Springer, 2007, 476 pages
- William J. Morokoff and Russel E. Caflisch, Quasi-Monte Carlo integration, J. Comput. Phys. 122 (1995), no. 2, 218--230. (At CiteSeer: (页面存档备份,存于))
- R. E. Caflisch, Monte Carlo and quasi-Monte Carlo methods, Acta Numerica vol. 7, Cambridge University Press, 1998, pp. 1-49.
- Josef Dick and Friedrich Pillichshammer, Digital Nets and Sequences. Discrepancy Theory and Quasi-Monte Carlo Integration, Cambridge University Press, Cambridge, 2010, ISBN 978-0-521-19159-3
- Michael Drmota and Robert F. Tichy, Sequences, discrepancies and applications, Lecture Notes in Math.,1651, Springer, Berlin, 1997, ISBN 3-540-62606-9
- William J. Morokoff and Russel E. Caflisch, Quasi-random sequences and their discrepancies, SIAM J. Sci. Comput. 15 (1994), no. 6, 1251--1279 (AtCiteSeer: (页面存档备份,存于))
- Harald Niederreiter. Random Number Generation and Quasi-Monte Carlo Methods. Society for Industrial and Applied Mathematics, 1992. ISBN 0-89871-295-5
- Harald G. Niederreiter, Quasi-Monte Carlo methods and pseudo-random numbers, Bull. Amer. Math. Soc. 84(1978), no. 6, 957--1041
- Oto Strauch and Štefan Porubský, Distribution of Sequences: A Sampler, Peter Lang Publishing House, Frankfurt am Main 2005, ISBN 3-631-54013-2
外部链接
- (英文)一个直观的拟蒙特卡罗方法简介