Part of a series on |
Artificial intelligence |
---|
![]() |
Artificial intelligence in healthcare is a term used to describe the use of machine-learning algorithms and software, or artificial intelligence (AI), to copy human cognition in the analysis, presentation, and understanding of complex medical and health care data, or to exceed human capabilities by providing new ways to diagnose, treat, or prevent disease.[1][2] Specifically, AI is the ability of computer algorithms to approximate conclusions based solely on input data.
The primary aim of health-related AI applications is to analyze relationships between clinical data and patient outcomes.[3] AI programs are applied to practices such as diagnostics, treatment protocol development, drug development, personalized medicine, and patient monitoring and care. What differentiates AI technology from traditional technologies in healthcare is the ability to gather larger and more diverse data, process it, and produce a well-defined output to the end-user. AI does this through machine learning algorithms and deep learning. Because radiographs are the most common imaging tests conducted in most radiology departments, the potential for AI to help with triage and interpretation of traditional radiographs (X-ray pictures) is particularly noteworthy.[4] These processes can recognize patterns in behavior and create their own logic. To gain useful insights and predictions, machine learning models must be trained using extensive amounts of input data. AI algorithms behave differently from humans in two ways: (1) algorithms are literal: once a goal is set, the algorithm learns exclusively from the input data and can only understand what it has been programmed to do, (2) and some deep learning algorithms are black boxes; algorithms can predict with extreme precision, but offer little to no comprehensible explanation to the logic behind its decisions aside from the data and type of algorithm used.[5]
As widespread use of AI in healthcare is relatively new, research is ongoing into its application in various fields of medicine and industry. Additionally, greater consideration is being given to the unprecedented ethical concerns related to its practice such as data privacy, automation of jobs, and representation biases.[6] Furthermore, new technologies brought about by AI in healthcare are often resisted by healthcare leaders, leading to slow and erratic adoption.[7]
History
Research in the 1960s and 1970s produced the first problem-solving program, or expert system, known as Dendral.[8][9] While it was designed for applications in organic chemistry, it provided the basis for a subsequent system MYCIN,[10] considered one of the most significant early uses of artificial intelligence in medicine.[10][11] MYCIN and other systems such as INTERNIST-1 and CASNET did not achieve routine use by practitioners, however.[12]
The 1980s and 1990s brought the proliferation of the microcomputer and new levels of network connectivity. During this time, there was a recognition by researchers and developers that AI systems in healthcare must be designed to accommodate the absence of perfect data and build on the expertise of physicians.[13] Approaches involving fuzzy set theory,[14] Bayesian networks,[15] and artificial neural networks,[16][17] have been applied to intelligent computing systems in healthcare.
Medical and technological advancements occurring over this half-century period that have enabled the growth of healthcare-related applications of AI to include:
- Improvements in computing power resulting in faster data collection and data processing[18]
- Growth of genomic sequencing databases[19]
- Widespread implementation of electronic health record systems[20]
- Improvements in natural language processing and computer vision, enabling machines to replicate human perceptual processes[21][22]
- Enhanced the precision of robot-assisted surgery[23]
- Increased tree-based machine learning models that allow flexibility in establishing health predictors[24]
- Improvements in deep learning techniques and data logs in rare diseases
AI algorithms can also be used to analyze large amounts of data through electronic health records for disease prevention and diagnosis. Medical institutions such as The Mayo Clinic, Memorial Sloan Kettering Cancer Center,[25][26] and the British National Health Service,[27] have developed AI algorithms for their departments. Large technology companies such as IBM[28] and Google,[27] have also developed AI algorithms for healthcare. Additionally, hospitals are looking to AI software to support operational initiatives that increase cost saving, improve patient satisfaction, and satisfy their staffing and workforce needs.[29] Currently, the United States government is investing billions of dollars to progress the development of AI in healthcare.[5] Companies are developing technologies that help healthcare managers improve business operations through increasing utilization, decreasing patient boarding, reducing length of stay and optimizing staffing levels.[30]
Clinical applications
Cardiovascular
Artificial intelligence algorithms have shown promising results in accurately diagnosing and risk stratifying patients with concern for coronary artery disease, showing potential as an initial triage tool.[31][32] Other algorithms have been used in predicting patient mortality, medication effects, and adverse events following treatment for acute coronary syndrome.[31] Wearables, smartphones, and internet-based technologies have also shown the ability to monitor patients' cardiac data points, expanding the amount of data and the various settings AI models can use and potentially enabling earlier detection of cardiac events occurring outside of the hospital.[33] Another growing area of research is the utility of AI in classifying heart sounds and diagnosing valvular disease.[34] Challenges of AI in cardiovascular medicine have included the limited data available to train machine learning models, such as limited data on social determinants of health as they pertain to cardiovascular disease.[35]
A key limitation in early studies evaluating AI were omissions of data comparing algorithmic performance to humans. Examples of studies which assess AI performance relative to physicians includes how AI is noninferior to humans in interpretation of cardiac echocardiograms[36] and that AI can diagnose heart attack better than human physicians in the emergency setting, reducing both low-value testing and missed diagnoses.[37]
In cardiovascular tissue engineering and organoid studies, AI is increasingly used to analyze microscopy images, and integrate electrophysiological read outs.[38]
Dermatology
Dermatology is an imaging abundant speciality[39] and the development of deep learning has been strongly tied to image processing. Therefore, there is a natural fit between the dermatology and deep learning. There are 3 main imaging types in dermatology: contextual images, macro images, micro images.[40] For each modality, deep learning showed great progress.[41] Han et al. showed keratinocytic skin cancer detection from face photographs.[42] Esteva et al. demonstrated dermatologist-level classification of skin cancer from lesion images.[43] Noyan et al. demonstrated a convolutional neural network that achieved 94% accuracy at identifying skin cells from microscopic Tzanck smear images.[44] A concern raised with this work is that it has not engaged with disparities related to skin color or differential treatment of patients with non-white skin tones.[45]
Recent advances have suggested the use of AI to describe and evaluate the outcome of maxillo-facial surgery or the assessment of cleft palate therapy in regard to facial attractiveness or age appearance.[46][47]
In 2018, a paper published in the journal Annals of Oncology mentioned that skin cancer could be detected more accurately by an artificial intelligence system (which used a deep learning convolutional neural network) than by dermatologists. On average, the human dermatologists accurately detected 86.6% of skin cancers from the images, compared to 95% for the CNN machine.[48]
Gastroenterology
AI can play a role in various facets of the field of gastroenterology. Endoscopic exams such as esophagogastroduodenoscopies (EGD) and colonoscopies rely on rapid detection of abnormal tissue. By enhancing these endoscopic procedures with AI, clinicians can more rapidly identify diseases, determine their severity, and visualize blind spots. Early trials in using AI detection systems of early gastric cancer have shown sensitivity close to expert endoscopists.[49]
Infectious diseases
AI has shown potential in both the laboratory and clinical spheres of infectious disease medicine.[50] As the novel coronavirus ravages through the globe, the United States is estimated to invest more than $2 billion in AI-related healthcare research by 2025, more than 4 times the amount spent in 2019 ($463 million).[51] While neural networks have been developed to rapidly and accurately detect a host response to COVID-19 from mass spectrometry samples, a scoping review of the literature found few examples of AI being used directly in clinical practice during the COVID-19 pandemic itself.[52] Other applications include support-vector machines identifying antimicrobial resistance, machine learning analysis of blood smears to detect malaria, and improved point-of-care testing of Lyme disease based on antigen detection. Additionally, AI has been investigated for improving diagnosis of meningitis, sepsis, and tuberculosis, as well as predicting treatment complications in hepatitis B and hepatitis C patients.[50]
Musculoskeletal
AI has been used to identify causes of knee pain that doctors miss, that disproportionately affect Black patients.[53] Underserved populations experience higher levels of pain. These disparities persist even after controlling for the objective severity of diseases like osteoarthritis, as graded by human physicians using medical images, raising the possibility that underserved patients’ pain stems from factors external to the knee, such as stress. Researchers have conducted a study using a machine-learning algorithm to show that standard radiographic measures of severity overlook objective but undiagnosed features that disproportionately affect diagnosis and management of underserved populations with knee pain. They proposed that new algorithmic measure ALG-P could potentially enable expanded access to treatments for underserved patients.[54]
Neurology
The use of AI technologies has been explored for use in the diagnosis and prognosis of Alzheimer's Disease. For diagnostic purposes, machine learning models have been developed that rely on structural MRI inputs.[55] The input datasets for these models are drawn from databases such as the Alzheimer's Disease Neuroimaging Initiative.[56] Researchers have developed models that rely on convolutional neural networks with the aim of improving early diagnostic accuracy.[57] Generative adversarial networks are a form of deep learning that have also performed well in diagnosing AD.[58] There have also been efforts to develop machine learning models into forecasting tools that can predict the prognosis of patients with AD. Forecasting patient outcomes through generative models has been proposed by researchers as a means of synthesizing training and validation sets.[59] They suggest that generated patient forecasts could be used to provide future models larger training datasets than current open access databases.
Oncology
AI has been explored for use in cancer diagnosis, risk stratification, molecular characterization of tumors, and cancer drug discovery. A particular challenge in oncologic care that AI is being developed to address is the ability to accurately predict which treatment protocols will be best suited for each patient based on their individual genetic, molecular, and tumor-based characteristics.[60] Through its ability to translate images to mathematical sequences, AI has been trialed in cancer diagnostics with the reading of imaging studies and pathology slides.[61]
In January 2020, researchers demonstrated an AI system, based on a Google DeepMind algorithm, capable of surpassing human experts in breast cancer detection.[62][63] In July 2020, it was reported that an AI algorithm developed by the University of Pittsburgh achieves the highest accuracy to date in identifying prostate cancer, with 98% sensitivity and 97% specificity.[64][65]
In 2023 a study reported the use of AI for CT-based radiomics classification at grading the aggressiveness of retroperitoneal sarcoma with 82% accuracy compared with 44% for lab analysis of biopsies.[66][67]
Ophthalmology
Artificial intelligence-enhanced technology is being used as an aid in the screening of eye disease and prevention of blindness.[68] In 2018, the U.S. Food and Drug Administration authorized the marketing of the first medical device to diagnose a specific type of eye disease, diabetic retinopathy using an artificial intelligence algorithm.[69] Moreover, AI technology may be used to further improve "diagnosis rates" because of the potential to decrease detection time.[70]
Pathology
For many diseases, pathological analysis of cells and tissues is considered to be the gold standard of disease diagnosis. Methods of digital pathology allows microscopy slides to be scanned and digitally analyzed. AI-assisted pathology tools have been developed to assist with the diagnosis of a number of diseases, including breast cancer, hepatitis B, gastric cancer, and colorectal cancer. AI has also been used to predict genetic mutations and prognosticate disease outcomes.[49] AI is well-suited for use in low-complexity pathological analysis of large-scale screening samples, such as colorectal or breast cancer screening, thus lessening the burden on pathologists and allowing for faster turnaround of sample analysis.[72] Several deep learning and artificial neural network models have shown accuracy similar to that of human pathologists,[72] and a study of deep learning assistance in diagnosing metastatic breast cancer in lymph nodes showed that the accuracy of humans with the assistance of a deep learning program was higher than either the humans alone or the AI program alone.[73] Additionally, implementation of digital pathology is predicted to save over $12 million for a university center over the course of five years,[74] though savings attributed to AI specifically have not yet been widely researched. The use of augmented and virtual reality could prove to be a stepping stone to wider implementation of AI-assisted pathology, as they can highlight areas of concern on a pathology sample and present them in real-time to a pathologist for more efficient review.[72] AI also has the potential to identify histological findings at levels beyond what the human eye can see,[72] and has shown the ability to use genotypic and phenotypic data to more accurately detect the tumor of origin for metastatic cancer.[75] One of the major current barriers to widespread implementation of AI-assisted pathology tools is the lack of prospective, randomized, multi-center controlled trials in determining the true clinical utility of AI for pathologists and patients, highlighting a current area of need in AI and healthcare research.[72]
Primary care
Primary care has become one key development area for AI technologies.[76][77] AI in primary care has been used for supporting decision making, predictive modelling, and business analytics.[78] There are only a few examples of AI decision support systems that were prospectively assessed on clinical efficacy when used in practice by physicians. But there are cases where the use of these systems yielded a positive effect on treatment choice by physicians.[79]
Psychiatry
In psychiatry, AI applications are still in a phase of proof-of-concept.[80] Areas where the evidence is widening quickly include predictive modelling of diagnosis and treatment outcomes,[81] chatbots, conversational agents that imitate human behaviour and which have been studied for anxiety and depression.[82]
Challenges include the fact that many applications in the field are developed and proposed by private corporations, such as the screening for suicidal ideation implemented by Facebook in 2017.[83] Such applications outside the healthcare system raise various professional, ethical and regulatory questions.[84] Another issue is often with the validity and interpretability of the models. Small training datasets contain bias that is inherited by the models, and compromises the generalizability and stability of these models. Such models may also have the potential to be discriminatory against minority groups that are underrepresented in samples.[85]
Radiology
AI is being studied within the field of radiology to detect and diagnose diseases through Computerized Tomography (CT) and Magnetic Resonance (MR) Imaging.[86] It may be particularly useful in settings where demand for human expertise exceeds supply, or where data is too complex to be efficiently interpreted by human readers.[87] Several deep learning models have shown the capability to be roughly as accurate as healthcare professionals in identifying diseases through medical imaging, though few of the studies reporting these findings have been externally validated.[88] AI can also provide non-interpretive benefit to radiologists, such as reducing noise in images, creating high-quality images from lower doses of radiation, enhancing MR image quality,[89] and automatically assessing image quality.[90] Further research investigating the use of AI in nuclear medicine focuses on image reconstruction, anatomical landmarking, and the enablement of lower doses in imaging studies.[91] The analysis of images for supervised AI applications in radiology encompasses two primary techniques at present: (1) convolutional neural network-based analysis; and (2) utilization of radiomics.[92]
Systems applications
Disease diagnosis
An article by Jiang, et al. (2017) demonstrated that there are several types of AI techniques that have been used for a variety of different diseases, such as support vector machines, neural networks, and decision trees. Each of these techniques is described as having a "training goal" so "classifications agree with the outcomes as much as possible…".
To demonstrate some specifics for disease diagnosis/classification there are two different techniques used in the classification of these diseases including using artificial neural networks (ANN) and Bayesian networks (BN). It was found that ANN was better and could more accurately classify diabetes and cardiovascular disease.
Through the use of machine learning classifiers (MLC's), artificial intelligence has been able to substantially aid doctors in patient diagnosis through the manipulation of mass electronic health records (EHR's).[93] Medical conditions have grown more complex, and with a vast history of electronic medical records building, the likelihood of case duplication is high.[93] Although someone today with a rare illness is less likely to be the only person to have had any given disease, the inability to access cases from similarly symptomatic origins is a major roadblock for physicians.[93] The implementation of AI to not only help find similar cases and treatments, such as through early predictors of Alzheimer's disease and dementias,[94] but also factor in chief symptoms and help the physicians ask the most appropriate questions helps the patient receive the most accurate diagnosis and treatment possible.[93]
Recent developments in statistical physics, machine learning, and inference algorithms are being explored for their potential in improving medical diagnostic approaches.[95] Combining the skills of medical professionals and machines can help overcome decision-making weaknesses in medical practice. To do so, one needs precise disease definitions and a probabilistic analysis of symptoms and molecular profiles. Physicists have been studying similar problems for years, using microscopic elements and their interactions to extract macroscopic states of various physical systems. Physics inspired machine learning approaches can thus be applied to study disease processes and to perform biomarker analysis.
Telemedicine
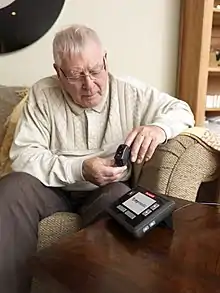
The increase of telemedicine, the treatment of patients remotely, has shown the rise of possible AI applications.[96] AI can assist in caring for patients remotely by monitoring their information through sensors.[97] A wearable device may allow for constant monitoring of a patient and the ability to notice changes that may be less distinguishable by humans. The information can be compared to other data that has already been collected using artificial intelligence algorithms that alert physicians if there are any issues to be aware of.[97]
Another application of artificial intelligence is chat-bot therapy. Some researchers charge that the reliance on chatbots for mental healthcare does not offer the reciprocity and accountability of care that should exist in the relationship between the consumer of mental healthcare and the care provider (be it a chat-bot or psychologist), though.[98]
Since the average age has risen due to a longer life expectancy, artificial intelligence could be useful in helping take care of older populations.[99] Tools such as environment and personal sensors can identify a person's regular activities and alert a caretaker if a behavior or a measured vital is abnormal.[99] Although the technology is useful, there are also discussions about limitations of monitoring in order to respect a person's privacy since there are technologies that are designed to map out home layouts and detect human interactions.[99]
Electronic health records
Electronic health records (EHR) are crucial to the digitalization and information spread of the healthcare industry. Now that around 80% of medical practices use EHR, the next step is to use artificial intelligence to interpret the records and provide new information to physicians.[100]
One application uses natural language processing (NLP) to make more succinct reports that limit the variation between medical terms by matching similar medical terms.[100] For example, the term heart attack and myocardial infarction mean the same things, but physicians may use one over the over based on personal preferences.[100] NLP algorithms consolidate these differences so that larger datasets can be analyzed.[100] Another use of NLP identifies phrases that are redundant due to repetition in a physician's notes and keeps the relevant information to make it easier to read.[100] Other applications use concept processing to analyze the information entered by the current patient's doctor to present similar cases and help the physician remember to include all relevant details.[101]
Beyond making content edits to an EHR, there are AI algorithms that evaluate an individual patient's record and predict a risk for a disease based on their previous information and family history.[102] One general algorithm is a rule-based system that makes decisions similarly to how humans use flow charts.[103] This system takes in large amounts of data and creates a set of rules that connect specific observations to concluded diagnoses.[103] Thus, the algorithm can take in a new patient's data and try to predict the likeliness that they will have a certain condition or disease.[103] Since the algorithms can evaluate a patient's information based on collective data, they can find any outstanding issues to bring to a physician's attention and save time.[102] One study conducted by the Centerstone research institute found that predictive modeling of EHR data has achieved 70–72% accuracy in predicting individualized treatment response.[104] These methods are helpful due to the fact that the amount of online health records doubles every five years.[102] Physicians do not have the bandwidth to process all this data manually, and AI can leverage this data to assist physicians in treating their patients.[102]
Drug interactions
Improvements in natural language processing led to the development of algorithms to identify drug-drug interactions in medical literature.[105][106][107][108] Drug-drug interactions pose a threat to those taking multiple medications simultaneously, and the danger increases with the number of medications being taken.[109] To address the difficulty of tracking all known or suspected drug-drug interactions, machine learning algorithms have been created to extract information on interacting drugs and their possible effects from medical literature. Efforts were consolidated in 2013 in the DDIExtraction Challenge, in which a team of researchers at Carlos III University assembled a corpus of literature on drug-drug interactions to form a standardized test for such algorithms.[110] Competitors were tested on their ability to accurately determine, from the text, which drugs were shown to interact and what the characteristics of their interactions were.[111] Researchers continue to use this corpus to standardize the measurement of the effectiveness of their algorithms.[105][106][108]
Other algorithms identify drug-drug interactions from patterns in user-generated content, especially electronic health records and/or adverse event reports.[106][107] Organizations such as the FDA Adverse Event Reporting System (FAERS) and the World Health Organization's VigiBase allow doctors to submit reports of possible negative reactions to medications. Deep learning algorithms have been developed to parse these reports and detect patterns that imply drug-drug interactions.[112]
Industry
The trend of large health companies merging allows for greater health data accessibility. Greater health data lays the groundwork for the implementation of AI algorithms.
A large part of industry focus of implementation of AI in the healthcare sector is in the clinical decision support systems. As more data is collected, machine learning algorithms adapt and allow for more robust responses and solutions.[86] Numerous companies are exploring the possibilities of the incorporation of big data in the healthcare industry. Many companies investigate the market opportunities through the realms of "data assessment, storage, management, and analysis technologies" which are all crucial parts of the healthcare industry.[113]
The following are examples of large companies that have contributed to AI algorithms for use in healthcare:
- IBM's Watson Oncology is in development at Memorial Sloan Kettering Cancer Center and Cleveland Clinic. IBM is also working with CVS Health on AI applications in chronic disease treatment and with Johnson & Johnson on analysis of scientific papers to find new connections for drug development. In May 2017, IBM and Rensselaer Polytechnic Institute began a joint project entitled Health Empowerment by Analytics, Learning and Semantics (HEALS), to explore using AI technology to enhance healthcare.
- Microsoft's Hanover project, in partnership with Oregon Health & Science University's Knight Cancer Institute, analyzes medical research to predict the most effective cancer drug treatment options for patients. Other projects include medical image analysis of tumor progression and the development of programmable cells.
- Google's DeepMind platform is being used by the UK National Health Service to detect certain health risks through data collected via a mobile app. A second project with the NHS involves the analysis of medical images collected from NHS patients to develop computer vision algorithms to detect cancerous tissues.
- Tencent is working on several medical systems and services. These include AI Medical Innovation System (AIMIS), an AI-powered diagnostic medical imaging service; WeChat Intelligent Healthcare; and Tencent Doctorwork
- Intel's venture capital arm Intel Capital recently invested in startup Lumiata which uses AI to identify at-risk patients and develop care options.
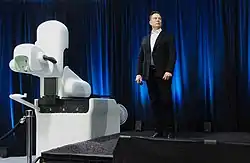
- Neuralink has come up with a next-generation neuroprosthetic which intricately interfaces with thousands of neural pathways in the brain.[86] Their process allows a chip, roughly the size of a quarter, to be inserted in the place of a chunk of a skull by a precision surgical robot to avoid accidental injury .[86]
Digital consultant apps use AI to give medical consultation based on personal medical history and common medical knowledge. Users report their symptoms into the app, which uses speech recognition to compare against a database of illnesses. Babylon then offers a recommended action, taking into account the user's medical history. Entrepreneurs in healthcare have been effectively using seven business model archetypes to take AI solution[buzzword] to the marketplace. These archetypes depend on the value generated for the target user (e.g. patient focus vs. healthcare provider and payer focus) and value capturing mechanisms (e.g. providing information or connecting stakeholders).
IFlytek launched a service robot "Xiao Man", which integrated artificial intelligence technology to identify the registered customer and provide personalized recommendations in medical areas. It also works in the field of medical imaging. Similar robots are also being made by companies such as UBTECH ("Cruzr") and Softbank Robotics ("Pepper").
The Indian startup Haptik recently developed a WhatsApp chatbot which answers questions associated with the deadly coronavirus in India.
With the market for AI expanding constantly, large tech companies such as Apple, Google, Amazon, and Baidu all have their own AI research divisions, as well as millions of dollars allocated for acquisition of smaller AI based companies.[113] Many automobile manufacturers are beginning to use machine learning healthcare in their cars as well.[113] Companies such as BMW, GE, Tesla, Toyota, and Volvo all have new research campaigns to find ways of learning a driver's vital statistics to ensure they are awake, paying attention to the road, and not under the influence of substances or in .[113]
Expanding care to developing nations
Artificial intelligence continues to expand in its abilities to diagnose more people accurately in nations where fewer doctors are accessible to the public. Many new technology companies such as SpaceX and the Raspberry Pi Foundation have enabled more developing countries to have access to computers and the internet than ever before.[114] With the increasing capabilities of AI over the internet, advanced machine learning algorithms can allow patients to get accurately diagnosed when they would previously have no way of knowing if they had a life-threatening disease or not.[114]
Using AI in developing nations that do not have the resources will diminish the need for outsourcing and can improve patient care. AI can allow for not only diagnosis of patient in areas where healthcare is scarce, but also allow for a good patient experience by resourcing files to find the best treatment for a patient.[115] The ability of AI to adjust course as it goes also allows the patient to have their treatment modified based on what works for them; a level of individualized care that is nearly non-existent in developing countries.[115]
Regulation
While research on the use of AI in healthcare aims to validate its efficacy in improving patient outcomes before its broader adoption, its use may nonetheless introduce several new types of risk to patients and healthcare providers, such as algorithmic bias, Do not resuscitate implications, and other machine morality issues. AI may also compromise the protection of patients' rights, such as the right to informed consent and the right to medical data protection.[116] These challenges of the clinical use of AI have brought about a potential need for regulations.
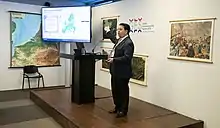
Currently, there are regulations pertaining to the collection of patient data. This includes policies such as the Health Insurance Portability and Accountability Act (HIPAA) and the European General Data Protection Regulation (GDPR).[117] The GDPR pertains to patients within the EU and details the consent requirements for patient data use when entities collect patient healthcare data. Similarly, HIPAA protects healthcare data from patient records in the United States.[117] In May 2016, the White House announced its plan to host a series of workshops and formation of the National Science and Technology Council (NSTC) Subcommittee on Machine Learning and Artificial Intelligence. In October 2016, the group published The National Artificial Intelligence Research and Development Strategic Plan, outlining its proposed priorities for Federally-funded AI research and development (within government and academia). The report notes a strategic R&D plan for the subfield of health information technology is in development stages.
The only agency that has expressed concern is the FDA. Bakul Patel, the Associate Center Director for Digital Health of the FDA, is quoted saying in May 2017:
"We're trying to get people who have hands-on development experience with a product's full life cycle. We already have some scientists who know artificial intelligence and machine learning, but we want complementary people who can look forward and see how this technology will evolve."
United Nations (WHO/ITU)
The joint ITU-WHO Focus Group on Artificial Intelligence for Health (FG-AI4H) has built a platform - known as the ITU-WHO AI for Health Framework - for the testing and benchmarking of AI applications in health domain. As of November 2018, eight use cases are being benchmarked, including assessing breast cancer risk from histopathological imagery, guiding anti-venom selection from snake images, and diagnosing skin lesions.
US FDA
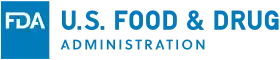
In January 2021, the US FDA published a new Action Plan, entitled Artificial Intelligence (AI) /Machine Learning (ML)-Based Software as a Medical Device (SaMD) Action Plan.[119] This plan lays out the FDA's future plans for regulation of medical devices that would include artificial intelligence in their software. There are five main actions the FDA plans to take to increase regulation: 1. Tailored Regulatory Framework for Ai/M:-based SaMD, 2. Good Machine Learning Practice (GMLP), 3. Patient-Centered Approach Incorporating Transparency to Users, 4. Regulatory Science Methods Related to Algorithm Bias & Robustness, and 5. Real-World Performance(RWP). This plan was in direct response to stakeholders' feedback on a 2019 discussion paper also published by the FDA.
According to the U.S. Department of Health and Human Services, the Office for Civil Rights (OCR) has issued guidance on the ethical use of AI in healthcare. The guidance outlines four core ethical principles that must be followed: respect for autonomy, beneficence, non-maleficence, and justice. Respect for autonomy requires that individuals have control over their own data and decisions. Beneficence requires that AI be used to do good, such as improving the quality of care and reducing health disparities. Non-maleficence requires that AI be used to do no harm, such as avoiding discrimination in decisions. Finally, justice requires that AI be used fairly, such as using the same standards for decisions no matter a person's race, gender, or income level. Moreover, as of March 2021, the OCR hired a Chief Artificial Intelligence Officer (OCAIO) to pursue the "implementation of the HHS AI strategy".[120] The OCR also has issued rules and regulations to protect the privacy of individuals’ health information. These regulations require healthcare providers to follow certain privacy rules when using AI. The OCR also requires healthcare providers to keep a record of how they use AI and to ensure that their AI systems are secure. Overall, the U.S. has taken steps to protect individuals’ privacy and ethical issues related to AI in healthcare[121]
The U.S. is not the only country to develop or initiate regulations of data privacy with AI. Other countries have implemented data protection regulations, more specifically with company privacy invasions. In Denmark, the Danish Expert Group on Data Ethics has adopted recommendations on 'Data for the Benefit of the People'. These recommendations are intended to encourage the responsible use of data in the business sector, with a focus on data processing. The recommendations include a focus on equality and non-discrimination with regard to bias in AI, as well as human dignity. The importance of human dignity is stressed, as it is said to outweigh profit and must be respected in all data processes[122]
The European Union has implemented the General Data Protection Regulation (GDPR) to protect citizens' personal data, which applies to the use of AI in healthcare. In addition, the European Commission has established guidelines to ensure the ethical development of AI, including the use of algorithms to ensure fairness and transparency.[123] With GDPR, the European Union was the first to regulate AI through data protection legislation. The Union finds privacy as a fundamental human right, it wants to prevent unconsented and secondary uses of data by private or public health facilities. By streamlining access to personal data for health research and findings, they are able to instate the right and importance of patient privacy.[123] In the United States, the Health Insurance Portability and Accountability Act (HIPAA) requires organizations to protect the privacy and security of patient information. The Centers for Medicare and Medicaid Services have also released guidelines for the development of AI-based medical applications.[124]
Ethical concerns
Data collection
In order to effectively train Machine Learning and use AI in healthcare, massive amounts of data must be gathered. Acquiring this data, however, comes at the cost of patient privacy in most cases and is not well received publicly. For example, a survey conducted in the UK estimated that 63% of the population is uncomfortable with sharing their personal data in order to improve artificial intelligence technology.[117] The scarcity of real, accessible patient data is a hindrance that deters the progress of developing and deploying more artificial intelligence in healthcare.
Automation
A systematic review and thematic analysis in 2023 showed that most stakeholders including health professionals, patients, and the general public doubted that care involving AI could be empathetic.[125]
According to a 2019 study, AI can replace up to 35% of jobs in the UK within the next 10 to 20 years.[126] However, of these jobs, it was concluded that AI has not eliminated any healthcare jobs so far. Though if AI were to automate healthcare-related jobs, the jobs most susceptible to automation would be those dealing with digital information, radiology, and pathology, as opposed to those dealing with doctor-to-patient interaction.[126]
Automation can provide benefits alongside doctors as well. It is expected that doctors who take advantage of AI in healthcare will provide greater quality healthcare than doctors and medical establishments who do not.[127] AI will likely not completely replace healthcare workers but rather give them more time to attend to their patients. AI may avert healthcare worker burnout and cognitive overload
AI will ultimately help contribute to the progression of societal goals which include better communication, improved quality of healthcare, and autonomy.[128]
Recently, there have been many discussions between healthcare experts in terms of AI and elder care. In relation to elder care, AI bots have been helpful in guiding older residents living in assisted living with entertainment and company. These bots are allowing staff in the home to have more one-on-one time with each resident, but the bots are also programmed with more ability in what they are able to do; such as knowing different languages and different types of care depending on the patient's conditions. The bot is an AI machine, which means it goes through the same training as any other machine - using algorithms to parse the given data, learn from it and predict the outcome in relation to what situation is at hand[129]
Bias
Since AI makes decisions solely on the data it receives as input, it is important that this data represents accurate patient demographics. In a hospital setting, patients do not have full knowledge of how predictive algorithms are created or calibrated. Therefore, these medical establishments can unfairly code their algorithms to discriminate against minorities and prioritize profits rather than providing optimal care.[130] A recent scoping review identified 18 equity challenges along with 15 strategies that can be implemented to help address them when AI applications are developed using many-to-many mapping.[131]
There can also be unintended bias in these algorithms that can exacerbate social and healthcare inequities.[130] Since AI's decisions are a direct reflection of its input data, the data it receives must have accurate representation of patient demographics. White males are overly represented in medical data sets.[132] Therefore, having minimal patient data on minorities can lead to AI making more accurate predictions for majority populations, leading to unintended worse medical outcomes for minority populations.[133] Collecting data from minority communities can also lead to medical discrimination. For instance, HIV is a prevalent virus among minority communities and HIV status can be used to discriminate against patients.[132] In addition to biases that may arise from sample selection, different clinical systems used to collect data may also impact AI functionality. For example, radiographic systems and their outcomes (e.g., resolution) vary by provider. Moreover, clinician work practices, such as the positioning of the patient for radiography, can also greatly influence the data and make comparability difficult.[134] However, these biases are able to be eliminated through careful implementation and a methodical collection of representative data.
A final source of bias, which has been called “label choice bias,” arises when proxy measures are used to train algorithms, that build in bias against certain groups. For example, a widely used algorithm predicted health care costs as a proxy for health care needs, and used predictions to allocate resources to help patients with complex health needs. This introduced bias because Black patients have lower costs, even when they are just as unhealthy as White patients.[135] Solutions to the "label choice bias" aim to match the actual target (what the algorithm is predicting) more closely to the ideal target (what researchers want the algorithm to predict), so for the prior example, instead of predicting cost, researchers would focus on the variable of healthcare needs which is rather more significant. Adjusting the target led to almost double the number of Black patients being selected for the program
See also
- AI alignment
- Artificial intelligence in mental health
- Artificial intelligence
- Glossary of artificial intelligence
- Full body scanner (i.e. Dermascanner, ...)
- BlueDot
- Clinical decision support system
- Computer-aided diagnosis
- Computer-aided simple triage
- Google DeepMind
- IBM Watson Health
- Medical image computing
- Michal Rosen-Zvi
- Speech recognition software in healthcare
- The MICCAI Society
References
- ↑ "Developing an aging clock using deep learning on retinal images". ai.googleblog.com. 2023-04-11. Retrieved 2023-06-01.
- ↑ Mullainathan S, Obermeyer Z (May 2022). "Solving medicine's data bottleneck: Nightingale Open Science". Nature Medicine. 28 (5): 897–899. doi:10.1038/s41591-022-01804-4. PMID 35534570. S2CID 248668494.
- ↑ Coiera E (1997). Guide to medical informatics, the Internet and telemedicine. Chapman & Hall, Ltd.
- ↑ Adams, Scott J.; Henderson, Robert D. E.; Yi, Xin; Babyn, Paul (February 2021). "Artificial Intelligence Solutions for Analysis of X-ray Images". Canadian Association of Radiologists Journal. 72 (1): 60–72. doi:10.1177/0846537120941671. ISSN 0846-5371. PMID 32757950. S2CID 221036912.
- 1 2 Luca M, Kleinberg J, Mullainathan S (January–February 2016). "Algorithms Need Managers, Too". Harvard Business Review. Retrieved 2018-10-08.
- ↑ Floridi L, Luetge C, Pagallo U, Schafer B, Valcke P, Vayena E, et al. (2019-09-01). "Key Ethical Challenges in the European Medical Information Framework". Minds and Machines. 29 (3): 355–371. doi:10.1007/s11023-018-9467-4. hdl:2318/1728336. ISSN 1572-8641. S2CID 49668711.
- ↑ Petersson L, Larsson I, Nygren JM, Nilsen P, Neher M, Reed JE, et al. (July 2022). "Challenges to implementing artificial intelligence in healthcare: a qualitative interview study with healthcare leaders in Sweden". BMC Health Services Research. 22 (1): 850. doi:10.1186/s12913-022-08215-8. PMC 9250210. PMID 35778736.
- ↑ Lindsay RK, Buchanan BG, Feigenbaum EA, Lederberg J (1993). "DENDRAL: a case study of the first expert system for scientific hypothesis formation". Artificial Intelligence. 61 (2): 209–261. doi:10.1016/0004-3702(93)90068-m. hdl:2027.42/30758.
- ↑ Lea, Andrew S. (2023). Digitizing Diagnosis: Medicine, Minds, and Machines in Twentieth-Century America. Johns Hopkins University Press. pp. 1–256. ISBN 978-1421446813.
- 1 2 Clancey WJ, Shortliffe EH (1984). Readings in medical artificial intelligence: the first decade. Addison-Wesley Longman Publishing Co., Inc.
- ↑ Bruce G, Buchanan BG, Shortliffe ED (1984). Rule-based expert systems: the MYCIN experiments of the Stanford Heuristic Programming Project.
- ↑ Duda RO, Shortliffe EH (April 1983). "Expert Systems Research". Science. 220 (4594): 261–268. Bibcode:1983Sci...220..261D. doi:10.1126/science.6340198. PMID 6340198.
- ↑ Miller RA (1994). "Medical diagnostic decision support systems--past, present, and future: a threaded bibliography and brief commentary". Journal of the American Medical Informatics Association. 1 (1): 8–27. doi:10.1136/jamia.1994.95236141. PMC 116181. PMID 7719792.
- ↑ Adlassnig KP (July 1980). "A fuzzy logical model of computer-assisted medical diagnosis". Methods of Information in Medicine. 19 (3): 141–148. doi:10.1055/s-0038-1636674. PMID 6997678.
- ↑ Reggia JA, Peng Y (September 1987). "Modeling diagnostic reasoning: a summary of parsimonious covering theory". Computer Methods and Programs in Biomedicine. 25 (2): 125–134. doi:10.1016/0169-2607(87)90048-4. PMC 2244953. PMID 3315427.
- ↑ Baxt WG (December 1991). "Use of an artificial neural network for the diagnosis of myocardial infarction". Annals of Internal Medicine. 115 (11): 843–848. doi:10.7326/0003-4819-115-11-843. PMID 1952470.
- ↑ Maclin PS, Dempsey J, Brooks J, Rand J (February 1991). "Using neural networks to diagnose cancer". Journal of Medical Systems. 15 (1): 11–19. doi:10.1007/bf00993877. PMID 1748845. S2CID 10189561.
- ↑ Koomey J, Berard S, Sanchez M, Wong H (March 2010). "Implications of historical trends in the electrical efficiency of computing". IEEE Annals of the History of Computing. 33 (3): 46–54. CiteSeerX 10.1.1.323.9505. doi:10.1109/MAHC.2010.28. S2CID 8305701.
- ↑ Barnes B, Dupré J (2009). Genomes and what to make of them. University of Chicago Press.
- ↑ Jha AK, DesRoches CM, Campbell EG, Donelan K, Rao SR, Ferris TG, et al. (April 2009). "Use of electronic health records in U.S. hospitals". The New England Journal of Medicine. 360 (16): 1628–1638. doi:10.1056/NEJMsa0900592. PMID 19321858. S2CID 19914056.
- ↑ Banko M, Brill E (July 2001). "Scaling to very very large corpora for natural language disambiguation" (PDF). Proceedings of the 39th Annual Meeting on Association for Computational Linguistics. Association for Computational Linguistics: 26–33. Archived from the original (PDF) on 2019-04-07. Retrieved 2019-04-07.
- ↑ Dougherty G (2009). Digital image processing for medical applications. Cambridge University Press.
- ↑ "Artificial Intelligence and Machine Learning for Healthcare". Sigmoidal. 21 December 2017. Archived from the original on 17 August 2020. Retrieved 26 March 2018.
- ↑ Hu L, Li L (December 2022). "Using Tree-Based Machine Learning for Health Studies: Literature Review and Case Series". International Journal of Environmental Research and Public Health. 19 (23): 16080. doi:10.3390/ijerph192316080. PMC 9736500. PMID 36498153.
- ↑ Power B (19 March 2015). "Artificial Intelligence Is Almost Ready for Business". Harvard Business Review. Massachusetts General Hospital.
- ↑ Bahl M, Barzilay R, Yedidia AB, Locascio NJ, Yu L, Lehman CD (March 2018). "High-Risk Breast Lesions: A Machine Learning Model to Predict Pathologic Upgrade and Reduce Unnecessary Surgical Excision". Radiology. 286 (3): 810–818. doi:10.1148/radiol.2017170549. PMID 29039725. S2CID 4910088.
- 1 2 Bloch-Budzier S (22 November 2016). "NHS using Google technology to treat patients". BBC News.
- ↑ Lorenzetti L (5 April 2016). "Here's How IBM Watson Health is Transforming the Health Care Industry". Fortune.
- ↑ Kent J (2018-08-08). "Providers Embrace Predictive Analytics for Clinical, Financial Benefits". HealthITAnalytics. Retrieved 2019-01-16.
- ↑ Lee K (4 January 2016). "Predictive analytics in healthcare helps improve OR utilization". SearchHealthIT. Retrieved 2019-01-16.
- 1 2 Wang H, Zu Q, Chen J, Yang Z, Ahmed MA (October 2021). "Application of Artificial Intelligence in Acute Coronary Syndrome: A Brief Literature Review". Advances in Therapy. 38 (10): 5078–5086. doi:10.1007/s12325-021-01908-2. PMID 34528221. S2CID 237522871.
- ↑ Infante T, Cavaliere C, Punzo B, Grimaldi V, Salvatore M, Napoli C (December 2021). "Radiogenomics and Artificial Intelligence Approaches Applied to Cardiac Computed Tomography Angiography and Cardiac Magnetic Resonance for Precision Medicine in Coronary Heart Disease: A Systematic Review". Circulation: Cardiovascular Imaging. 14 (12): 1133–1146. doi:10.1161/CIRCIMAGING.121.013025. PMID 34915726. S2CID 245284764.
- ↑ Sotirakos S, Fouda B, Mohamed Razif NA, Cribben N, Mulhall C, O'Byrne A, et al. (February 2022). "Harnessing artificial intelligence in cardiac rehabilitation, a systematic review". Future Cardiology. 18 (2): 154–164. doi:10.2217/fca-2021-0010. PMID 33860679. S2CID 233258636.
- ↑ Chen W, Sun Q, Chen X, Xie G, Wu H, Xu C (May 2021). "Deep Learning Methods for Heart Sounds Classification: A Systematic Review". Entropy. 23 (6): 667. Bibcode:2021Entrp..23..667C. doi:10.3390/e23060667. PMC 8229456. PMID 34073201.
- ↑ Zhao Y, Wood EP, Mirin N, Cook SH, Chunara R (October 2021). "Social Determinants in Machine Learning Cardiovascular Disease Prediction Models: A Systematic Review". American Journal of Preventive Medicine. 61 (4): 596–605. doi:10.1016/j.amepre.2021.04.016. PMID 34544559.
- ↑ He B, Kwan AC, Cho JH, Yuan N, Pollick C, Shiota T, et al. (April 2023). "Blinded, randomized trial of sonographer versus AI cardiac function assessment". Nature. 616 (7957): 520–524. Bibcode:2023Natur.616..520H. doi:10.1038/s41586-023-05947-3. PMC 10115627. PMID 37020027.
- ↑ Mullainathan S, Obermeyer Z (2022). ""Diagnosing Physician Error: A Machine Learning Approach to Low-Value Health Care"". The Quarterly Journal of Economics. 137 (2): 679–727. doi:10.1093/qje/qjab046 – via Oxford Academic.
- ↑ Bax, Monique; Thorpe, Jordan; Romanov, Valentin (December 2023). "The future of personalized cardiovascular medicine demands 3D and 4D printing, stem cells, and artificial intelligence". Frontiers in Sensors. 4. doi:10.3389/fsens.2023.1294721. ISSN 2673-5067.
- ↑ Hibler BP, Qi Q, Rossi AM (March 2016). "Current state of imaging in dermatology". Seminars in Cutaneous Medicine and Surgery. 35 (1): 2–8. doi:10.12788/j.sder.2016.001. PMID 26963110.
- ↑ "Image acquisition in dermatology | DermNet NZ". dermnetnz.org. Retrieved 2021-02-23.
- ↑ Chan S, Reddy V, Myers B, Thibodeaux Q, Brownstone N, Liao W (June 2020). "Machine Learning in Dermatology: Current Applications, Opportunities, and Limitations". Dermatology and Therapy. 10 (3): 365–386. doi:10.1007/s13555-020-00372-0. PMC 7211783. PMID 32253623.
- ↑ Han SS, Moon IJ, Lim W, Suh IS, Lee SY, Na JI, et al. (January 2020). "Keratinocytic Skin Cancer Detection on the Face Using Region-Based Convolutional Neural Network". JAMA Dermatology. 156 (1): 29–37. doi:10.1001/jamadermatol.2019.3807. PMC 6902187. PMID 31799995.
- ↑ Esteva A, Kuprel B, Novoa RA, Ko J, Swetter SM, Blau HM, Thrun S (February 2017). "Dermatologist-level classification of skin cancer with deep neural networks". Nature. 542 (7639): 115–118. Bibcode:2017Natur.542..115E. doi:10.1038/nature21056. PMC 8382232. PMID 28117445. S2CID 3767412.
- ↑ Noyan MA, Durdu M, Eskiocak AH (October 2020). "TzanckNet: a convolutional neural network to identify cells in the cytology of erosive-vesiculobullous diseases". Scientific Reports. 10 (1): 18314. doi:10.1038/s41598-020-75546-z. PMC 7591506. PMID 33110197.
- ↑ Adamson AS, Smith A (November 2018). "Machine Learning and Health Care Disparities in Dermatology". JAMA Dermatology. 154 (11): 1247–1248. doi:10.1001/jamadermatol.2018.2348. PMID 30073260. S2CID 51907489.
- ↑ Patcas R, Bernini DA, Volokitin A, Agustsson E, Rothe R, Timofte R (January 2019). "Applying artificial intelligence to assess the impact of orthognathic treatment on facial attractiveness and estimated age". International Journal of Oral and Maxillofacial Surgery. 48 (1): 77–83. doi:10.1016/j.ijom.2018.07.010. PMID 30087062.
- ↑ Patcas R, Timofte R, Volokitin A, Agustsson E, Eliades T, Eichenberger M, Bornstein MM (August 2019). "Facial attractiveness of cleft patients: a direct comparison between artificial-intelligence-based scoring and conventional rater groups". European Journal of Orthodontics. 41 (4): 428–433. doi:10.1093/ejo/cjz007. hdl:20.500.11850/377316. PMID 30788496. S2CID 73507799.
- ↑ "Computer learns to detect skin cancer more accurately than doctors". The Guardian. 29 May 2018.
- 1 2 Cao JS, Lu ZY, Chen MY, Zhang B, Juengpanich S, Hu JH, et al. (April 2021). "Artificial intelligence in gastroenterology and hepatology: Status and challenges". World Journal of Gastroenterology. 27 (16): 1664–1690. doi:10.3748/wjg.v27.i16.1664. PMC 8072192. PMID 33967550.
- 1 2 Tran NK, Albahra S, May L, Waldman S, Crabtree S, Bainbridge S, Rashidi H (December 2021). "Evolving Applications of Artificial Intelligence and Machine Learning in Infectious Diseases Testing". Clinical Chemistry. 68 (1): 125–133. doi:10.1093/clinchem/hvab239. PMC 9383167. PMID 34969102.
- ↑ "COVID-19 Pandemic Impact: Global R&D Spend For AI in Healthcare and Pharmaceuticals Will Increase US$1.5 Billion By 2025". Medical Letter on the CDC & FDA. May 3, 2020 – via Gale Academic OneFile.
- ↑ Mann S, Berdahl CT, Baker L, Girosi F (October 2022). Pani D (ed.). "Artificial intelligence applications used in the clinical response to COVID-19: A scoping review". PLOS Digital Health. 1 (10): e0000132. doi:10.1371/journal.pdig.0000132. PMC 9931281. PMID 36812557.
- ↑ "AI could make health care fairer—by helping us believe what patients say". MIT Technology Review. Retrieved 2023-06-03.
- ↑ Pierson E, Cutler DM, Leskovec J, Mullainathan S, Obermeyer Z (January 2021). "An algorithmic approach to reducing unexplained pain disparities in underserved populations". Nature Medicine. 27 (1): 136–140. doi:10.1038/s41591-020-01192-7. PMID 33442014. S2CID 256839494.
- ↑ Khojaste-Sarakhsi M, Shahabi-Haghighi S, Fatemi-Ghomi S, Marchiori E (August 2022). "Deep learning for Alzheimer's disease diagnosis: A survey". Artificial Intelligence in Medicine. 130 (933–3657). doi:10.1016/j.artmed.2022.102332. PMID 35809971. S2CID 249633553.
- ↑ "About ADNI". 2017. Retrieved October 30, 2023.
- ↑ Nguyen HD, Clément M, Mansencal B, Coupé P (21 September 2021). Deep Grading Based on Collective Artificial Intelligence for AD Diagnosis and Prognosis. Interpretability of Machine Intelligence in Medical Image Computing, and Topological Data Analysis and Its Applications for Medical Data. Springer, Cham. pp. 24–33. arXiv:2211.15192. doi:10.1007/978-3-030-87444-5_3.
- ↑ Qu C, Zou Y, Ma Y, Chen Q, Luo J, Fan H, Jia Z, Gong Q, Chen T (21 April 2022). "Diagnostic Performance of Generative Adversarial Network-Based Depp Learning Methods for Alzheimer's Disease: A Systematic Review and Meta-Analysis". Frontiers in Aging Neuroscience. 14. doi:10.3389/fnagi.2022.841696. PMC 9068970. PMID 35527734.
- ↑ Fisher Charles K, Smith Aaron M, Walsh Jonathan R, Coalition Against Major Diseases (2019). "Machine Learning for comprehensive forecasting of Alzheimer's Disease progression". Scientific Reports. 9 (1): 13622. arXiv:1807.03876. Bibcode:2019NatSR...913622F. doi:10.1038/s41598-019-49656-2. PMC 6754403. PMID 31541187.
- ↑ Bhinder B, Gilvary C, Madhukar NS, Elemento O (April 2021). "Artificial Intelligence in Cancer Research and Precision Medicine". Cancer Discovery. 11 (4): 900–915. doi:10.1158/2159-8290.CD-21-0090. PMC 8034385. PMID 33811123.
- ↑ Majumder A, Sen D (October 2021). "Artificial intelligence in cancer diagnostics and therapy: current perspectives". Indian Journal of Cancer. 58 (4): 481–492. doi:10.4103/ijc.IJC_399_20. PMID 34975094. S2CID 240522128.
- ↑ Kobie N (1 January 2020). "DeepMind's new AI can spot breast cancer just as well as your doctor". Wired. Retrieved 1 January 2020.
- ↑ McKinney SM, Sieniek M, Godbole V, Godwin J, Antropova N, Ashrafian H, et al. (January 2020). "International evaluation of an AI system for breast cancer screening". Nature. 577 (7788): 89–94. Bibcode:2020Natur.577...89M. doi:10.1038/s41586-019-1799-6. hdl:10044/1/76203. PMID 31894144. S2CID 209523468.
- ↑ "Artificial intelligence identifies prostate cancer with near-perfect accuracy". EurekAlert!. 27 July 2020. Retrieved 29 July 2020.
- ↑ Pantanowitz L, Quiroga-Garza GM, Bien L, Heled R, Laifenfeld D, Linhart C, et al. (August 2020). "An artificial intelligence algorithm for prostate cancer diagnosis in whole slide images of core needle biopsies: a blinded clinical validation and deployment study". The Lancet. Digital Health. 2 (8): e407–e416. doi:10.1016/S2589-7500(20)30159-X. PMID 33328045.
- ↑ Arthur, Amani; Orton, Matthew R; Emsley, Robby; Vit, Sharon; Kelly-Morland, Christian; Strauss, Dirk; Lunn, Jason; Doran, Simon; Lmalem, Hafida; Nzokirantevye, Axelle; Litiere, Saskia; Bonvalot, Sylvie; Haas, Rick; Gronchi, Alessandro; Van Gestel, Dirk (November 2023). "A CT-based radiomics classification model for the prediction of histological type and tumour grade in retroperitoneal sarcoma (RADSARC-R): a retrospective multicohort analysis". The Lancet Oncology. 24 (11): 1277–1286. doi:10.1016/S1470-2045(23)00462-X. PMC 10618402. PMID 37922931.
- ↑ "Scientists excited by AI tool that grades severity of rare cancer". BBC News. 2023-11-01. Retrieved 2023-11-12.
- ↑ Ravindran S (April 2019). "How artificial intelligence is helping to prevent blindness". Nature. doi:10.1038/d41586-019-01111-y. PMID 32269369. S2CID 146005413.
- ↑ Office of the Commissioner (2020-03-24). "FDA permits marketing of artificial intelligence-based device to detect certain diabetes-related eye problems". FDA. Retrieved 2023-02-11.
- ↑ Han JH (August 2022). "Artificial Intelligence in Eye Disease: Recent Developments, Applications, and Surveys". Diagnostics. 12 (8): 1927. doi:10.3390/diagnostics12081927. PMC 9406878. PMID 36010277.
- ↑ Lourenço BC, Guimarães-Teixeira C, Flores BC, Miranda-Gonçalves V, Guimarães R, Cantante M, et al. (February 2022). "Ki67 and LSD1 Expression in Testicular Germ Cell Tumors Is Not Associated with Patient Outcome: Investigation Using a Digital Pathology Algorithm". Life. 12 (2): 264. Bibcode:2022Life...12..264L. doi:10.3390/life12020264. PMC 8875543. PMID 35207551.
- Figure 2 - available via license: Creative Commons Attribution 4.0 International - 1 2 3 4 5 Försch S, Klauschen F, Hufnagl P, Roth W (March 2021). "Artificial Intelligence in Pathology". Deutsches Ärzteblatt International. 118 (12): 194–204. doi:10.3238/arztebl.m2021.0011. PMC 8278129. PMID 34024323.
- ↑ Steiner DF, MacDonald R, Liu Y, Truszkowski P, Hipp JD, Gammage C, et al. (December 2018). "Impact of Deep Learning Assistance on the Histopathologic Review of Lymph Nodes for Metastatic Breast Cancer". The American Journal of Surgical Pathology. 42 (12): 1636–1646. doi:10.1097/PAS.0000000000001151. PMC 6257102. PMID 30312179.
- ↑ Ho J, Ahlers SM, Stratman C, Aridor O, Pantanowitz L, Fine JL, et al. (2014). "Can digital pathology result in cost savings? A financial projection for digital pathology implementation at a large integrated health care organization". Journal of Pathology Informatics. 5 (1): 33. doi:10.4103/2153-3539.139714. PMC 4168664. PMID 25250191.
- ↑ Jurmeister P, Bockmayr M, Seegerer P, Bockmayr T, Treue D, Montavon G, et al. (September 2019). "Machine learning analysis of DNA methylation profiles distinguishes primary lung squamous cell carcinomas from head and neck metastases". Science Translational Medicine. 11 (509): eaaw8513. doi:10.1126/scitranslmed.aaw8513. PMID 31511427. S2CID 202564269.
- ↑ Mistry P (September 2019). "Artificial intelligence in primary care". The British Journal of General Practice. 69 (686): 422–423. doi:10.3399/bjgp19X705137. PMC 6715470. PMID 31467001.
- ↑ Blease C, Kaptchuk TJ, Bernstein MH, Mandl KD, Halamka JD, DesRoches CM (March 2019). "Artificial Intelligence and the Future of Primary Care: Exploratory Qualitative Study of UK General Practitioners' Views". Journal of Medical Internet Research. 21 (3): e12802. doi:10.2196/12802. PMC 6446158. PMID 30892270. S2CID 59175658.
- ↑ Liyanage H, Liaw ST, Jonnagaddala J, Schreiber R, Kuziemsky C, Terry AL, de Lusignan S (August 2019). "Artificial Intelligence in Primary Health Care: Perceptions, Issues, and Challenges". Yearbook of Medical Informatics. 28 (1): 41–46. doi:10.1055/s-0039-1677901. PMC 6697547. PMID 31022751.
- ↑ Herter WE, Khuc J, Cinà G, Knottnerus BJ, Numans ME, Wiewel MA, et al. (May 2022). "Impact of a Machine Learning-Based Decision Support System for Urinary Tract Infections: Prospective Observational Study in 36 Primary Care Practices". JMIR Medical Informatics. 10 (5): e27795. doi:10.2196/27795. PMC 9118012. PMID 35507396. S2CID 246819392.
- ↑ Graham S, Depp C, Lee EE, Nebeker C, Tu X, Kim HC, Jeste DV (November 2019). "Artificial Intelligence for Mental Health and Mental Illnesses: an Overview". Current Psychiatry Reports. 21 (11): 116. doi:10.1007/s11920-019-1094-0. PMC 7274446. PMID 31701320.
- ↑ Chekroud AM, Bondar J, Delgadillo J, Doherty G, Wasil A, Fokkema M, et al. (June 2021). "The promise of machine learning in predicting treatment outcomes in psychiatry". World Psychiatry. 20 (2): 154–170. doi:10.1002/wps.20882. PMC 8129866. PMID 34002503.
- ↑ Fulmer R, Joerin A, Gentile B, Lakerink L, Rauws M (December 2018). "Using Psychological Artificial Intelligence (Tess) to Relieve Symptoms of Depression and Anxiety: Randomized Controlled Trial". JMIR Mental Health. 5 (4): e64. doi:10.2196/mental.9782. PMC 6315222. PMID 30545815.
- ↑ Coppersmith G, Leary R, Crutchley P, Fine A (January 2018). "Natural Language Processing of Social Media as Screening for Suicide Risk". Biomedical Informatics Insights. 10: 1178222618792860. doi:10.1177/1178222618792860. PMC 6111391. PMID 30158822.
- ↑ Brunn M, Diefenbacher A, Courtet P, Genieys W (August 2020). "The Future is Knocking: How Artificial Intelligence Will Fundamentally Change Psychiatry". Academic Psychiatry. 44 (4): 461–466. doi:10.1007/s40596-020-01243-8. PMID 32424706. S2CID 218682746.
- ↑ Rutledge RB, Chekroud AM, Huys QJ (April 2019). "Machine learning and big data in psychiatry: toward clinical applications". Current Opinion in Neurobiology. Machine Learning, Big Data, and Neuroscience. 55: 152–159. doi:10.1016/j.conb.2019.02.006. PMID 30999271. S2CID 115202826.
- 1 2 3 4 Pisarchik AN, Maksimenko VA, Hramov AE (October 2019). "From Novel Technology to Novel Applications: Comment on "An Integrated Brain-Machine Interface Platform With Thousands of Channels" by Elon Musk and Neuralink". Journal of Medical Internet Research. 21 (10): e16356. doi:10.2196/16356. PMC 6914250. PMID 31674923. S2CID 207818415.
- ↑ Hosny A, Parmar C, Quackenbush J, Schwartz LH, Aerts HJ (August 2018). "Artificial intelligence in radiology". Nature Reviews. Cancer. 18 (8): 500–510. doi:10.1038/s41568-018-0016-5. PMC 6268174. PMID 29777175.
- ↑ Liu X, Faes L, Kale AU, Wagner SK, Fu DJ, Bruynseels A, et al. (October 2019). "A comparison of deep learning performance against health-care professionals in detecting diseases from medical imaging: a systematic review and meta-analysis". The Lancet. Digital Health. 1 (6): e271–e297. doi:10.1016/S2589-7500(19)30123-2. PMID 33323251. S2CID 204037561.
- ↑ Georgescu MI, Ionescu RT, Miron AI, Savencu O, Ristea NC, Verga N, Khan FS (2023). Multimodal Multi-Head Convolutional Attention With Various Kernel Sizes for Medical Image Super-Resolution (Report). IEEE/CVF Winter Conference on Applications of Computer Vision. pp. 2195–2205.
- ↑ Richardson ML, Garwood ER, Lee Y, Li MD, Lo HS, Nagaraju A, et al. (September 2021). "Noninterpretive Uses of Artificial Intelligence in Radiology". Academic Radiology. 28 (9): 1225–1235. doi:10.1016/j.acra.2020.01.012. PMID 32059956.
- ↑ Seifert R, Weber M, Kocakavuk E, Rischpler C, Kersting D (March 2021). "Artificial Intelligence and Machine Learning in Nuclear Medicine: Future Perspectives". Seminars in Nuclear Medicine. 51 (2): 170–177. doi:10.1053/j.semnuclmed.2020.08.003. PMID 33509373. S2CID 224863373.
- ↑ Hosny, Ahmed; Parmar, Chintan; Quackenbush, John; Schwartz, Lawrence H.; Aerts, Hugo J. W. L. (August 2018). "Artificial intelligence in radiology". Nature Reviews Cancer. 18 (8): 500–510. doi:10.1038/s41568-018-0016-5. ISSN 1474-1768. PMC 6268174. PMID 29777175.
- 1 2 3 4 Liang H, Tsui BY, Ni H, Valentim CC, Baxter SL, Liu G, et al. (March 2019). "Evaluation and accurate diagnoses of pediatric diseases using artificial intelligence". Nature Medicine. 25 (3): 433–438. doi:10.1038/s41591-018-0335-9. PMID 30742121. S2CID 59945159.
- ↑ Li Q, Yang X, Xu J, Guo Y, He X, Hu H, et al. (February 2023). "Early prediction of Alzheimer's disease and related dementias using real-world electronic health records". Alzheimer's & Dementia. 19 (8): 3506–3518. doi:10.1002/alz.12967. PMID 36815661. S2CID 257101613.
- ↑ Ramezanpour A, Beam AL, Chen JH, Mashaghi A (November 2020). "Statistical Physics for Medical Diagnostics: Learning, Inference, and Optimization Algorithms". Diagnostics. Basel, Switzerland. 10 (11): 972. doi:10.3390/diagnostics10110972. PMC 7699346. PMID 33228143.
- ↑ Hamet P, Tremblay J (April 2017). "Artificial intelligence in medicine". Metabolism. Insights Into the Future of Medicine: Technologies, Concepts, and Integration. 69S: S36–S40. doi:10.1016/j.metabol.2017.01.011. PMID 28126242.
- 1 2 Pivovarov R, Elhadad N (September 2015). "Automated methods for the summarization of electronic health records". Journal of the American Medical Informatics Association. 22 (5): 938–947. doi:10.1093/jamia/ocv032. PMC 4986665. PMID 25882031. S2CID 1452.
- ↑ Kretzschmar K, Tyroll H, Pavarini G, Manzini A, Singh I (2020-08-19). "Can Your Phone Be Your Therapist? Young People's Ethical Perspectives on the Use of Fully Automated Conversational Agents (Chatbots) in Mental Health Support". Biomedical Informatics Insights. 11 (1–2): 35–44. doi:10.1177/2057047320950636. PMC 7441483.
- 1 2 3 Pouke M, Häkkilä J (December 2013). "Elderly healthcare monitoring using an avatar-based 3D virtual environment". International Journal of Environmental Research and Public Health. 10 (12): 7283–7298. doi:10.3390/ijerph10127283. PMC 3881167. PMID 24351747. S2CID 18535954.
- 1 2 3 4 5 Ferrante FE (June 2005). "Evolving telemedicine/ehealth technology". Telemedicine Journal and e-Health. 11 (3): 370–383. doi:10.1089/tmj.2005.11.370. PMID 16035932.
- ↑ Garza-Galicia, M., Sosa, M., Low, R., & Simini, F. (2017). Enseñanza de un Programa de Ortesis Mental Clínica: Experiencia con Estudiantes de Medicina en Uruguay. Memorias Del Congreso Nacional De Ingeniería Biomédica, 4(1), 91–94. Retrieved from: http://memorias.somib.org.mx/index.php/memorias/article/view/221 Archived 2021-08-13 at the Wayback Machine
- 1 2 3 4 Medrano IH, Guijarro JT, Belda C, Ureña A, Salcedo I, Anke LE, Saggion H (2018). "Savana: Re-using Electronic Health Records with Artificial Intelligence". International Journal of Interactive Multimedia and Artificial Intelligence. 4 (7): 8–12. doi:10.9781/ijimai.2017.03.001. ISSN 1989-1660.
- 1 2 3 Eren A, Subasi A, Coskun O (February 2008). "A decision support system for telemedicine through the mobile telecommunications platform". Journal of Medical Systems. 32 (1): 31–35. doi:10.1007/s10916-007-9104-x. PMID 18333403. S2CID 11082133.
- ↑ Bennett CC, Doub TW, Selove R (April 2012). "EHRs connect research and practice: Where predictive modeling, artificial intelligence, and clinical decision support intersect". Health Policy and Technology. 1 (2): 105–114. arXiv:1204.4927. doi:10.1016/j.hlpt.2012.03.001. ISSN 2211-8837. S2CID 25022446.
- 1 2 Bokharaeian B, Diaz A, Chitsaz H (2016). "Enhancing Extraction of Drug-Drug Interaction from Literature Using Neutral Candidates, Negation, and Clause Dependency". PLOS ONE. 11 (10): e0163480. Bibcode:2016PLoSO..1163480B. doi:10.1371/journal.pone.0163480. PMC 5047471. PMID 27695078.
- 1 2 3 Cai R, Liu M, Hu Y, Melton BL, Matheny ME, Xu H, et al. (February 2017). "Identification of adverse drug-drug interactions through causal association rule discovery from spontaneous adverse event reports". Artificial Intelligence in Medicine. 76: 7–15. doi:10.1016/j.artmed.2017.01.004. PMC 6438384. PMID 28363289.
- 1 2 Christopoulou F, Tran TT, Sahu SK, Miwa M, Ananiadou S (January 2020). "Adverse drug events and medication relation extraction in electronic health records with ensemble deep learning methods". Journal of the American Medical Informatics Association. 27 (1): 39–46. doi:10.1093/jamia/ocz101. PMC 6913215. PMID 31390003.
- 1 2 Zhou D, Miao L, He Y (May 2018). "Position-aware deep multi-task learning for drug-drug interaction extraction" (PDF). Artificial Intelligence in Medicine. 87: 1–8. doi:10.1016/j.artmed.2018.03.001. PMID 29559249. S2CID 3951020.
- ↑ García JS (2013-04-14). Optimización del tratamiento de enfermos pluripatológicos en atención primaria UCAMI HHUU Virgen del Rocio (Report). Sevilla. Spain – via ponencias de la II Reunión de Paciente Pluripatológico y Edad Avanzada Archived.
- ↑ Herrero-Zazo M, Segura-Bedmar I, Martínez P, Declerck T (October 2013). "The DDI corpus: an annotated corpus with pharmacological substances and drug-drug interactions". Journal of Biomedical Informatics. 46 (5): 914–920. doi:10.1016/j.jbi.2013.07.011. hdl:10016/20207. PMID 23906817.
- ↑ Segura Bedmar I, Martínez P, Herrero Zazo M (June 2013). Semeval-2013 task 9: Extraction of drug-drug interactions from biomedical texts (ddiextraction 2013). Second Joint Conference on Lexical and Computational Semantics. Vol. 2. Association for Computational Linguistics. pp. 341–350.
- ↑ Xu B, Shi X, Yin Y, Zhao Z, Zheng W, Lin H, et al. (July 2019). "Incorporating User Generated Content for Drug Drug Interaction Extraction Based on Full Attention Mechanism". IEEE Transactions on NanoBioscience. 18 (3): 360–367. doi:10.1109/TNB.2019.2919188. PMID 31144641. S2CID 169038906.
- 1 2 3 4 Quan XI, Sanderson J (December 2018). "Understanding the Artificial Intelligence Business Ecosystem". IEEE Engineering Management Review. 46 (4): 22–25. doi:10.1109/EMR.2018.2882430. ISSN 0360-8581. S2CID 59525052.
- 1 2 Abou-Zahra S, Brewer J, Cooper M (2018-04-23). "Artificial Intelligence (AI) for Web Accessibility". Proceedings of the 15th International Web for All Conference. W4A '18. Lyon, France: Association for Computing Machinery. pp. 1–4. doi:10.1145/3192714.3192834. ISBN 978-1-4503-5651-0. S2CID 49863409.
- 1 2 Guo J, Li B (2018-08-01). "The Application of Medical Artificial Intelligence Technology in Rural Areas of Developing Countries". Health Equity. 2 (1): 174–181. doi:10.1089/heq.2018.0037. PMC 6110188. PMID 30283865.
- ↑ Van Kolfschooten H (2022-02-01). "EU regulation of artificial intelligence: Challenges for patients' rights" (PDF). Common Market Law Review. 59 (1): 81–112. doi:10.54648/COLA2022005. ISSN 0165-0750. S2CID 248591427.
- 1 2 3 Lacassie E, Marquet P, Martin-Dupont S, Gaulier JM, Lachâtre G (September 2000). "A non-fatal case of intoxication with foxglove, documented by means of liquid chromatography-electrospray-mass spectrometry". Journal of Forensic Sciences. 45 (5): 1154–1158. doi:10.1520/JFS14845J. PMID 11005196.
- ↑ Office of the Commissioner (2022-11-30). "About FDA". FDA. Retrieved 2023-04-08.
- ↑ "Artificial Intelligence and Machine Learning in Software as a Medical Device". FDA. 2023-10-20.
- ↑ "HHS Artificial Intelligence (AI) Strategy". www.hhs.gov. 2021-12-22. Retrieved 2023-11-25.
- ↑ Office for Civil Rights (OCR) (2015-09-05). "Civil Rights for Providers of Health Care and Human Services". HHS.gov. Retrieved 2022-12-17.
- ↑ "Cambridge Core - Journals & Books Online | Cambridge University Press". Cambridge Core. Retrieved 2022-12-17.
- 1 2 Forcier MB (16 September 2019). ""Integrating Artificial Intelligence into Health Care through Data Access: Can the GDPR Act as a Beacon for Policymakers?"". Journal of Law and the Biosciences. 6 (1): 317–335. doi:10.1093/jlb/lsz013. PMC 6813940. PMID 31666972.
- ↑ "Sharing and Utilizing Health Data for AI Applications - Hhs.gov".
- ↑ Vo V, Chen G, Aquino YS, Carter SM, Do QN, Woode ME (November 2023). "Multi-stakeholder preferences for the use of artificial intelligence in healthcare: A systematic review and thematic analysis". Social Science & Medicine. 338 (1): 116357. doi:10.1016/j.socscimed.2023.116357. PMID 37949020.
- 1 2 Davenport T, Kalakota R (June 2019). "The potential for artificial intelligence in healthcare". Future Healthcare Journal. 6 (2): 94–98. doi:10.7861/futurehosp.6-2-94. PMC 6616181. PMID 31363513.
- ↑ U.S News Staff (2018-09-20). "Artificial Intelligence Continues to Change Health Care". US News.
- ↑ "AI for Health CareArtificial Intelligence for Health Care". GrayRipples.com | AI | iOS | Android | PowerApps. 2020-03-04. Retrieved 2020-11-04.
- ↑ Savage N (2022-01-19). "Robots rise to meet the challenge of caring for old people". Nature. 601 (7893): S8–S10. Bibcode:2022Natur.601S...8S. doi:10.1038/d41586-022-00072-z. PMID 35046591. S2CID 246064292.
- 1 2 Baric-Parker J, Anderson EE (November 2020). "Patient Data-Sharing for AI: Ethical Challenges, Catholic Solutions". The Linacre Quarterly. 87 (4): 471–481. doi:10.1177/0024363920922690. PMC 7551527. PMID 33100395.
- ↑ Berdahl CT, Baker L, Mann S, Osoba O, Girosi F (2023-02-07). "Strategies to Improve the Impact of Artificial Intelligence on Health Equity: Scoping Review". JMIR AI. 2: e42936. doi:10.2196/42936. ISSN 2817-1705. S2CID 256681439.
- 1 2 Nordling L (September 2019). "A fairer way forward for AI in health care". Nature. 573 (7775): S103–S105. Bibcode:2019Natur.573S.103N. doi:10.1038/d41586-019-02872-2. PMID 31554993. S2CID 202749329.
- ↑ Reddy S, Allan S, Coghlan S, Cooper P (March 2020). "A governance model for the application of AI in health care". Journal of the American Medical Informatics Association. 27 (3): 491–497. doi:10.1093/jamia/ocz192. PMC 7647243. PMID 31682262.
- ↑ Pumplun L, Fecho M, Wahl N, Peters F, Buxmann P (October 2021). "Adoption of Machine Learning Systems for Medical Diagnostics in Clinics: Qualitative Interview Study". Journal of Medical Internet Research. 23 (10): e29301. doi:10.2196/29301. PMC 8556641. PMID 34652275. S2CID 238990562.
- ↑ Evans M, Mathews AW (2019-10-24). "Researchers Find Racial Bias in Hospital Algorithm". Wall Street Journal. ISSN 0099-9660. Retrieved 2023-06-03.
Further reading
- Bennett CC, Doub TW, Selove R (June 2012). "EHRs connect research and practice: Where predictive modeling, artificial intelligence, and clinical decision support intersect". Health Policy and Technology. 1 (2): 105–14. arXiv:1204.4927. doi:10.1016/j.hlpt.2012.03.001. S2CID 25022446.
- Wahl B, Cossy-Gantner A, Germann S, Schwalbe NR (2018). "Artificial intelligence (AI) and global health: how can AI contribute to health in resource-poor settings?". BMJ Global Health. 3 (4): e000798. doi:10.1136/bmjgh-2018-000798. PMC 6135465. PMID 30233828.
- de Jesus A (21 November 2019). "Artificial Intelligence in Epidemiology". Emerj. AI Research and Advisory Company.
- "Accelerating America's Leadership in Artificial Intelligence". whitehouse.gov. 11 February 2019 – via National Archives.
- Wong J, Horwitz MM, Zhou L, Toh S (December 2018). "Using machine learning to identify health outcomes from electronic health record data". Current Epidemiology Reports. Springer Science and Business Media LLC. 5 (4): 331–342. doi:10.1007/s40471-018-0165-9. PMC 6289196. PMID 30555773.
- Lin WC, Chen JS, Chiang MF, Hribar MR (February 2020). "Applications of Artificial Intelligence to Electronic Health Record Data in Ophthalmology". Translational Vision Science & Technology. Association for Research in Vision and Ophthalmology (ARVO). 9 (2): 13. doi:10.1167/tvst.9.2.13. PMC 7347028. PMID 32704419.
- Moehring RW, Phelan M, Lofgren E, Nelson A, Dodds Ashley E, Anderson DJ, Goldstein BA (March 2021). "Development of a Machine Learning Model Using Electronic Health Record Data to Identify Antibiotic Use Among Hospitalized Patients". JAMA Network Open. American Medical Association (AMA). 4 (3): e213460. doi:10.1001/jamanetworkopen.2021.3460. PMC 8008288. PMID 33779743.
- Schwartz JT, Gao M, Geng EA, Mody KS, Mikhail CM, Cho SK (December 2019). "Applications of Machine Learning Using Electronic Medical Records in Spine Surgery". Neurospine. The Korean Spinal Neurosurgery Society. 16 (4): 643–653. doi:10.14245/ns.1938386.193. PMC 6945000. PMID 31905452.
- Shin S, Austin PC, Ross HJ, Abdel-Qadir H, Freitas C, Tomlinson G, et al. (February 2021). "Machine learning vs. conventional statistical models for predicting heart failure readmission and mortality". ESC Heart Failure. Wiley. 8 (1): 106–115. doi:10.1002/ehf2.13073. PMC 7835549. PMID 33205591.
- Cho SM, Austin PC, Ross HJ, Abdel-Qadir H, Chicco D, Tomlinson G, et al. (August 2021). "Machine Learning Compared With Conventional Statistical Models for Predicting Myocardial Infarction Readmission and Mortality: A Systematic Review". The Canadian Journal of Cardiology. Elsevier. 37 (8): 1207–1214. doi:10.1016/j.cjca.2021.02.020. PMID 33677098. S2CID 232141652.